Exclusive: the most-cited papers of the twenty-first century
A Nature analysis reveals the 25 highest-cited papers published this century and explores why they are breaking records
The first quarter of the twenty-first century has produced some huge scientific breakthroughs, ranging from the first mRNA vaccines and CRISPR-based gene-editing techniques to the discovery of the Higgs boson and the first measurements of gravitational waves. But you won’t find any of these advances described in the top-cited papers published since 2000.
That is one of the findings of an analysis by Nature’s news team of the 25 most-cited papers published in the twenty-first century. The articles garnering the most citations report developments in artificial intelligence (AI); approaches to improve the quality of research or systematic reviews; cancer statistics; and research software. However, a pioneering 2004 paper on experiments with graphene1 — work that won its authors the Nobel Prize in Physics in 2010 — is also among the twenty-first century’s most-cited.
Citations — the means by which authors acknowledge previous sources in the literature — are one measure of a paper’s influence. But the most highly cited papers generally aren’t the most famous scientific discoveries. Rather, these works tend to describe scientific methods or software, the workhorses on which scientists depend. “Scientists say they value methods, theory and empirical discoveries, but in practice the methods get cited more,” notes Misha Teplitskiy, a sociologist at the University of Michigan in Ann Arbor who studies how scholars cite work.
There are multiple databases tracking academic citations, but they look at different document sets and differ in citation numbers. Nature selected five databases for its analysis and took the median rankings across them. The databases cover tens of millions of papers published in the twenty-first century.
By this methodology, the top-cited paper of the twenty-first century is a 2016 report2 by researchers at tech giant Microsoft about “deep residual learning” networks, or ResNets. These are a type of artificial neural network — the neural-inspired algorithms that underpin deep learning and the AI advances that followed. The paper describes a way to train networks that have about 150 layers, roughly 5 times more than researchers were used to. The ResNet architecture solved the problem of signals dissipating when they travelled through many layers. The work was first published as a preprint in late 2015, when Microsoft researchers announced that they had aced an image-recognition competition using the approach.
The concept behind ResNets was one of the factors leading to AI tools that could play board games (AlphaGo), predict protein structure (AlphaFold) and eventually model language (ChatGPT). Before them, “deep learning was not that deep”, says one of the paper’s authors, Kaiming He, who now works at the Massachusetts Institute of Technology in Cambridge.
Microsoft’s paper isn’t universally held to be the highest-cited. According to the search engine Google Scholar, the creators of which sent a top-cited list to Nature, the paper is second, with 254,000 citations. And according to the Web of Science, a private database owned by US firm Clarivate that indexes a more limited selection of journals, it is third, with just over 100,000. In the five databases selected, Microsoft’s publication was highest-cited in two, second in two others and third in one — giving it the top median rank across all five (see ‘Top ten most-cited works of the twenty-first century’; a full list of the most-cited works is in the Supplementary information).
Top ten most-cited works of the twenty-first century
Rank (median) | Citation | Times cited (range across databases) |
---|---|---|
1 | Deep residual learning for image recognition (2016, preprint 2015) | 103,756–254,074 |
2 | Analysis of relative gene expression data using real-time quantitative PCR and the 2–ΔΔCT method (2001) | 149,953–185,480 |
3 | Using thematic analysis in psychology (2006) | 100,327–230,391 |
4 | Diagnostic and Statistical Manual of Mental Disorders, DSM-5 (2013) | 98,312–367,800 |
5 | A short history of SHELX (2007) | 76,523–99,470 |
6 | Random forests (2001) | 31,809–146,508 |
7 | Attention is all you need (2017) | 56,201–150,832 |
8 | ImageNet classification with deep convolutional neural networks (2017) | 46,860–137,997 |
9 | Global cancer statistics 2020: GLOBOCAN estimates of incidence and mortality worldwide for 36 cancers in 185 countries (2020) | 75,634–99,390 |
10 | Global cancer statistics 2018: GLOBOCAN estimates of incidence and mortality worldwide for 36 cancers in 185 countries (2016) | 66,844–93,433 |
Source databases: Web of Science, Scopus, OpenAlex, Dimensions, Google Scholar. For a list of the top 25 most-cited works, see Supplementary information
The Microsoft paper isn’t just a hit among papers published this century. It also appears in the top ten of all-time citations, according to a separate analysis conducted for Nature (see ‘These are the most-cited research papers of all time’).
Comparing citation counts is fraught with unfairness and inconsistencies. For instance, because the Microsoft work was published a decade ago, it has had more time than younger papers to accrue citations. It’s also in a field — computer science — that is seeing high volumes of research. Nature commissioned bibliometricians to do an analysis that controls for some of these factors, but, perhaps because only articles with extreme citation counts are being considered, similar papers remained on top, with only recent, heavily cited papers on the COVID-19 pandemic entering the lists (see Supplementary information).
Here, Nature explores some of the works that have become twenty-first-century citation greats.
The rise of AI
AI papers come with natural advantages in the citation stakes, notes Geoff Hinton, a computer scientist at the University of Toronto in Canada who won a share of the Nobel Prize in Physics last year for his work in AI. Papers in this area are relevant to a huge number of fields, and the twenty-first century has seen extremely rapid progress and a large volume of papers, he says.
Many people credit the deep-learning revolution, in which multi-layered artificial neural networks revealed their wide usefulness, to the work described in a paper3 Hinton co-authored in 2012. It presented a network (later named AlexNet) that beat other methods by a wide margin in a competition to recognize and label objects in images. That paper is number 8 on the twenty-first-century list, and a review paper4 on deep learning by Hinton and his co-authors is number 16. (The 2009 paper5 ‘ImageNet: A Large-Scale Hierarchical Image Database’, which presented the data sets that researchers used to train classification software, is number 24.)
Three years after the publication describing AlexNet, an influential paper reported modifications to the network’s architecture, resulting in U-nets, which require less training data to process images6. It’s now ranked 12th in the list. Co-author Olaf Ronneberger, a researcher who was recruited by Google DeepMind in London as a result of the publication, says the conference that eventually accepted the paper almost rejected it for not being novel enough. “It is still the main workhorse in most diffusion models for image generation,” he says.
Hot on the heels of that work came Microsoft’s now top-cited paper. And in 2017, researchers at Google published a landmark paper7 titled ‘Attention is all you need’, which presented the neural-network architecture known as a transformer. This underlies the advances in large language models that power tools such as ChatGPT by efficiently implementing a mechanism called self-attention, which lets networks prioritize relevant information when learning patterns. That paper is this century’s seventh-highest cited.
The open-source nature of much of the early academic work in machine learning has also boosted its citations, some researchers say. The sixth-most-cited paper, titled ‘Random forests’8, presents a machine-learning algorithm that improved on previous, similar methods. Statistician Adele Cutler at Utah State University in Logan worked with its late author, US statistician Leo Breiman, to extend the method. She says the paper is popular because the method is open-source, free and easy to use. It also works extremely well off the shelf, with little or no customization required.
Many of the AI papers were released as preprints before formal peer review, which complicates efforts to count their citations. Most commercial databases either don’t track preprints or don’t try to merge their citations with those of the final peer-reviewed article, meaning that real citation counts are higher. As it becomes more popular to cite preprints, databases might need to adopt new conventions for aggregating such mentions, says Paul Wouters, a retired scientometrics researcher at Leiden University in the Netherlands.
The OpenAlex database (one of Nature’s sources for this article) does attempt to aggregate mentions by merging preprint and final article, says co-founder Jason Priem at OurResearch, a non-profit scholarly services firm in Vancouver, Canada, which developed the database. And Google Scholar tries to group all versions of a work and aggregate citations to it, says co-founder Anurag Acharya, who works for Google in Mountain View, California.
Research software
The second-most-cited paper of the twenty-first century did not end up on the list by coincidence: it was written explicitly to give researchers something to cite.
About 25 years ago, pharmaceutical scientist Thomas Schmittgen submitted a paper that included data from a technique called quantitative PCR, which allows researchers to quantify the amount of DNA in a sample. To analyse his data, Schmittgen used equations found in a technical manual. “One of the reviewers came back and said, ‘You can’t cite a user manual in a paper,’” he says. So Schmittgen, now at the University of Florida in Gainesville, contacted the creator of the equations and together they published a paper9 that could be cited.
And cited it was: more than 162,000 times, according to the Web of Science. That is enough to send Schmittgen’s paper into the top ten of all-time-cited research.
Schmittgen’s paper is popular because its formulae provided a simple way for biologists to calculate changes in gene activity in response to different conditions, such as before and after treatment with a drug. A software program called DESeq2, described in a paper10 that makes the list at number 18, is also used to calculate changes in gene activity, but uses RNA sequencing data to do so.
Another highly cited software paper on the list (at number five) was written by British chemist George Sheldrick, who died in February this year. He created the SHELX suite of computer programs to analyse the scattering patterns of X-rays after they are shot through crystals of molecules, with the aim of revealing the molecules’ atomic structure. When he began the work in the 1970s, “my job was to teach chemistry, and I wrote the programs as a hobby in my spare time”, he told Nature a decade ago. In 2008, he wrote a review paper11 that he suggested should be cited when any SHELX programs were used; that work has now been cited some 70,000–90,000 times, depending on the database consulted.
Cancer and health research
Three top-cited papers are familiar fare in the introduction sections of cancer research papers. Two of them (numbers nine and ten) are the 2018 and 2020 reports by GLOBOCAN12,13, a World Health Organization project that tracks global cancer statistics every one or two years. GLOBOCAN data are used by researchers, advocates and policymakers who need to give the incidence or mortality rate for a specific cancer type, says Freddie Bray, lead author of the papers and a cancer epidemiologist at the International Agency for Research on Cancer in Lyons, France.
The third cancer paper (number 19) is a review14 that attempts to distil the complexity of cancer into a handful of characteristics commonly found in tumours. These “hallmarks of cancer” have helped to shape the field, says co-author Douglas Hanahan, a researcher at the Ludwig Institute for Cancer Research in Lausanne, Switzerland.
“Students have come up to me to say, ‘I’m studying cancer research because of this review,’” he says. “I feel like a rock star.”
At number four on the list is what is sometimes called ‘psychiatry’s bible’: the fifth edition of the Diagnostic and Statistical Manual of Mental Disorders (DSM-5), which was published in 2013, nearly 20 years after the previous iteration. The book15 describes the criteria for categorizing and diagnosing mental disorders including addiction and depression and is extensively used by researchers and health professionals worldwide. DSM-5 is the only book that Nature included in the list, because most of the databases in the analysis recorded it.
Improving research quality
Psychologists Virginia Braun and Victoria Clarke were used to getting only a handful of citations on their papers about gender and sexuality. So they watched with astonishment as their 2006 paper16 became the third-most-cited article published this century. “It has a life of its own,” Clarke says.
The pair wrote the article in 2005, when Braun was on sabbatical at the University of the West of England in Bristol, UK, where Clarke works. For years, they had been trying to teach students ‘thematic analysis’, a qualitative research approach used to explore patterns that address a question from a collection of data, such as interviews. Braun and Clarke noticed that the approach was poorly defined and described. Sometimes, authors would state that ‘themes emerged’ — as if rather mysteriously — from their research, and there was a sense that anything goes. That year, Braun and Clarke wrote a readable paper aimed at students that explained thematic analysis and included a checklist of criteria for doing it well.
After the paper’s publication, researchers started referring to thematic analysis — as outlined by Braun and Clarke — as the method they used, which sent its citation count off the charts. The paper has “been completely life-changing”, says Clarke. She and Braun have since pivoted much of their work to thematic analysis and have received invitations to meetings across the world. “It was entirely accidental,” adds Braun, who works at the University of Auckland in New Zealand.
Enjoying our latest content?
Login or create an account to continue
- Access the most recent journalism from Nature's award-winning team
- Explore the latest features & opinion covering groundbreaking research
or
Sign in or create an account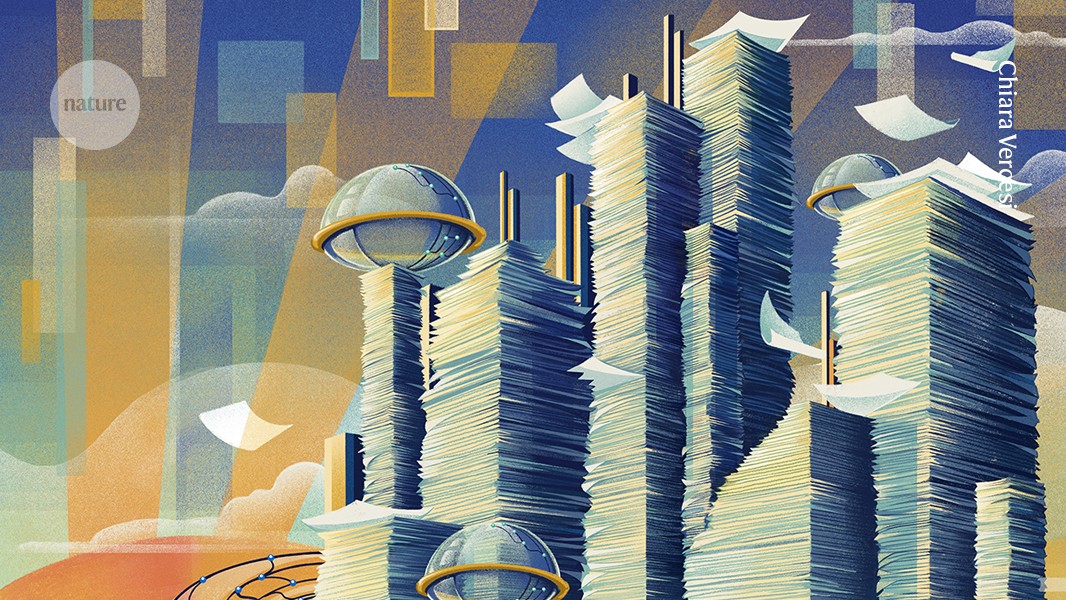
Nature 640, 588-592 (2025)
doi: https://doi.org/10.1038/d41586-025-01125-9
This story originally appeared on: Nature - Author:Helen Pearson