More-powerful AI is coming. Academia and industry must oversee it — together The safest and best results will come when academic and industry scientists collaborate to guide its development

AI companies want to give machines human-level intelligence, or AGI
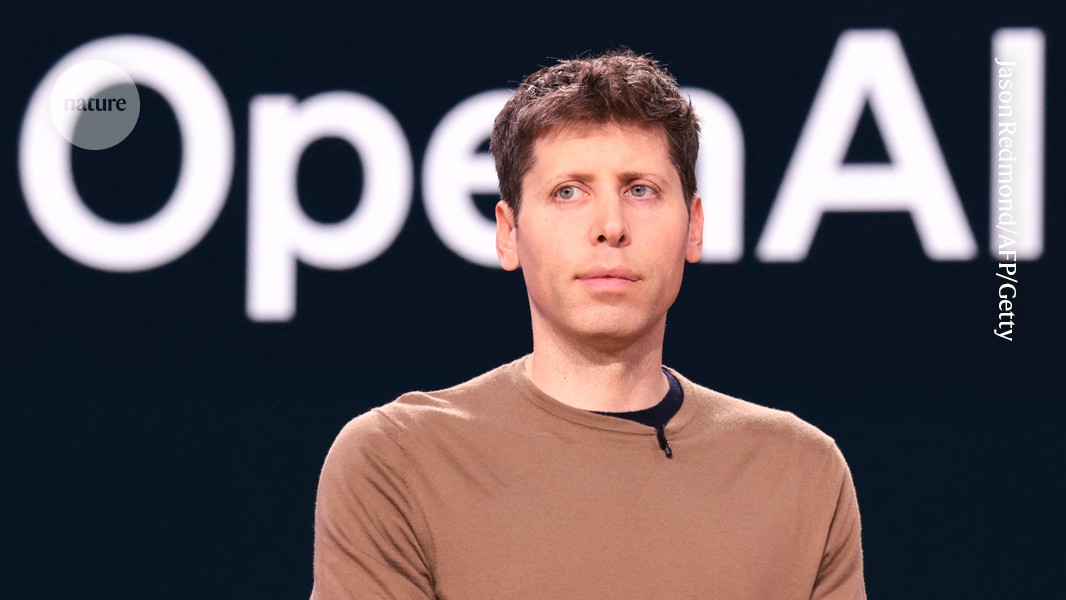
Sam Altman of OpenAI, the company that developed ChatGPT, says that machines will create superintelligence. Research is needed to verify these and other claims.Credit: Jason Redmond/AFP/Getty
“It is possible that we will have superintelligence in a few thousand days (!); it may take longer, but I’m confident we’ll get there.”
So wrote Sam Altman, the chief executive of OpenAI, a technology firm based in San Francisco, California, on 23 September. This was less than two weeks after the company behind ChatGPT released o1, its most advanced large language model (LLM) yet. Once confined to the realms of science fiction, the rise of LLMs in the past few years has renewed the relevance of the question of when we might create artificial general intelligence (AGI). Although it lacks a precise definition, AGI broadly refers to an AI system capable of human-level reasoning, generalization, planning and autonomy.
Policymakers everywhere have questions about AGI, including what its benefits and risks will be. These are not easy questions to answer, especially given that much of the work is happening in the private sector, in which studies are not always published openly. But what is clear is that AI companies are laser-focused on giving their systems the whole range of cognitive abilities enjoyed by humans. Companies developing AI models have a strong incentive to maintain the idea that AGI is nigh, to attract interest and therefore investment.
There was a consensus among researchers who spoke to Nature for a News Feature published this week (see Nature 636, 22–25; 2024) that large language models (LLMs), such as o1, Google’s Gemini and Claude, made by Anthropic, based in San Francisco, have not yet delivered AGI. And, informed by insights from neuroscience, many say that there are good reasons to think that LLMs never will, and that another technology will be needed for AI to achieve human-level intelligence.
Despite their breadth of ability — from generating computer code to summarizing academic articles and answering mathematics questions — there are fundamental limitations in the way the most powerful LLMs work, which involves essentially devouring a mass of data and using that to predict the next ‘token’ in a series. That generates plausible answers to a problem, rather than actually solving it.
François Chollet, a software engineer formerly at Google, based in Mountain View, California, and Subbarao Kambhampati, a computer scientist at Arizona State University in Tempe, have tested o1’s performance on tasks that require abstract reasoning and planning, and found that it comes up short of AGI. If AGI is to happen, some researchers think that AI systems would need coherent ‘world models’, or representations of their surroundings that they can use to test hypotheses, reason, plan and generalize knowledge learnt in one domain to potentially limitless other situations.
This is where ideas from neuroscience and cognitive science could propel the next breakthroughs. Yoshua Bengio’s team at the University of Montreal, Canada, for example, is exploring alternative AI architectures that would better support the building of coherent world models and the ability to reason using such models.
Some researchers argue that the next breakthroughs in AI might come not from the biggest systems, but from smaller, more-energy-efficient AI. Smarter systems in the future could also require less data to train if they had the ability to decide which aspects of their environment to sample, rather than simply ingesting everything they are fed, says Karl Friston, a theoretical neuroscientist at University College London.
Such work demonstrates that researchers from an array of fields need to be involved in AI development. This will be necessary to verify what systems are truly capable of, ensure that they live up to the technology companies’ claims and identify the breakthroughs needed for development. However, right now, accessing the leading AI systems can be difficult for researchers who don’t work at the companies that can afford the vast quantity of graphical processing units (GPUs) needed to train the systems (A. Khandelwal et al. Preprint at arXiv https://doi.org/nt67; 2024).
To give a sense of the scale of activity, in 2021, US government agencies (excluding the Department of Defense) allocated US$1.5 billion to AI research and development, and the European Commission spends around €1 billion (US$1.05 billion) annually. By contrast, companies worldwide spent more than $340 billion on AI research in 2021 (N. Ahmed et al. Science 379, 884–886; 2023). There are ways that governments could fund AI research on a bigger scale, for example by pooling resources. The Confederation of Laboratories for Artificial Intelligence Research in Europe, a non-profit organization based in The Hague, the Netherlands, has suggested building a ‘CERN for AI’ that can attract the same level of talent as AI companies do and so create a cutting-edge research environment.
It is hard to predict when AGI might arrive — estimates range from a few years from now to a decade or longer. But more huge advances in AI will certainly happen, and many of them will probably come from industry, considering the scale of investment. To ensure that these advances are beneficial, the research from technology companies needs to be verified using the best current understanding of what constitutes human intelligence, according to neuroscience, cognitive science, social science and other relevant fields. This publicly funded research needs to have a key role in AGI’s development.
Humanity needs to bring all knowledge to bear so that applications of AI research are robust and its risks are mitigated as much as possible. Governments, companies, research funders and researchers need to recognize their complementary strengths. If they do not, then insights that could help to improve AI will be missed — and the resulting systems risk being unpredictable and therefore unsafe.
doi: https://doi.org/10.1038/d41586-024-03911-3
This story originally appeared on: Nature - Author:furtherReadingSection