Has your paper been used to train an AI model? Almost certainly

Artificial-intelligence developers are buying access to valuable data sets that contain research papers — raising uncomfortable questions about copyright
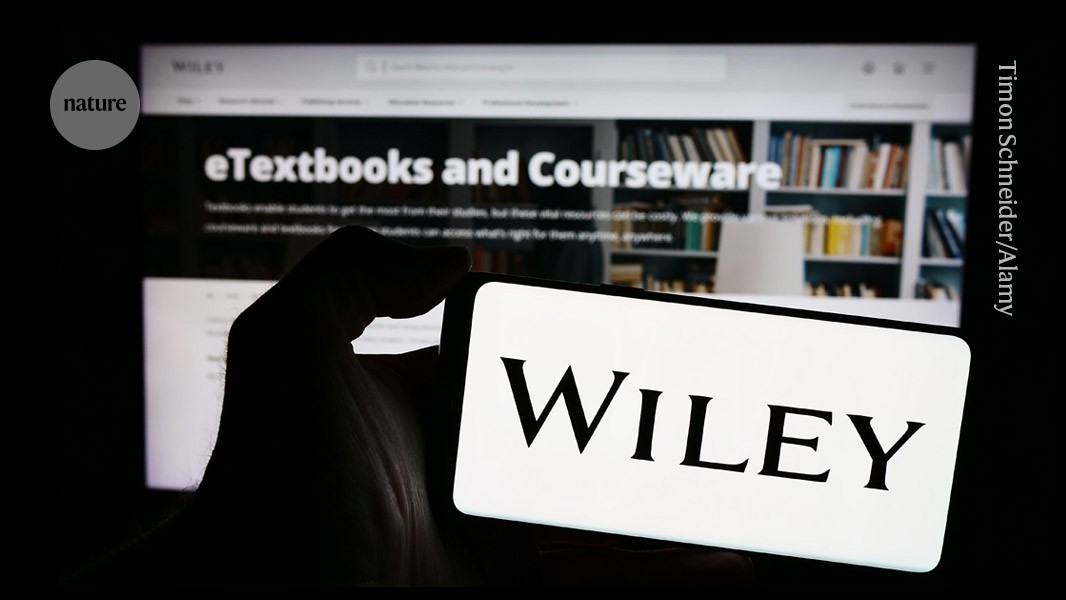
Academic publisher Wiley has sold access to its research papers to firms developing large language models.Credit: Timon Schneider/Alamy
Academic publishers are selling access to research papers to technology firms to train artificial-intelligence (AI) models. Some researchers have reacted with dismay at such deals happening without the consultation of authors. The trend is raising questions about the use of published and sometimes copyrighted work to train the exploding number of AI chatbots in development.
Experts say that, if a research paper hasn’t yet been used to train a large language model (LLM), it probably will be soon. Researchers are exploring technical ways for authors to spot if their content being used.
Last month, it emerged that the UK academic publisher Taylor & Francis, had signed a US$10-million deal with Microsoft, allowing the US technology company to access the publisher’s data to improve its AI systems. And in June, an investor update showed that US publisher Wiley had earned $23 million from allowing an unnamed company to train generative-AI models on its content.
Anything that is available to read online — whether in an open-access repository or not — is “pretty likely” to have been fed into an LLM already, says Lucy Lu Wang, an AI researcher at the University of Washington in Seattle. “And if a paper has already been used as training data in a model, there’s no way to remove that paper after the model has been trained,” she adds.
Massive data sets
LLMs train on huge volumes of data, frequently scraped from the Internet. They derive patterns between the often billions of snippets of language in the training data, known as tokens, that allow them to generate text with uncanny fluency.
Generative-AI models rely on absorbing patterns from these swathes of data to output text, images or computer code. Academic papers are valuable for LLM builders owing to their length and “high information density”, says Stefan Baack, who analyses AI training data sets at the Mozilla Foundation, a global non-profit organization in San Francisco, California that aims to keep the Internet open for all to access.
Training models on a large body of scientific information also give them a much better ability to reason about scientific topics, says Wang, who co-created S2ORC, a data set based on 81.1 million academic papers. The data set was originally developed for text mining — applying analytical techniques to find patterns in data — but has since been used to train LLMs.
The trend of buying high-quality data sets is growing. This year, the Financial Times has offered its content to ChatGPT developer OpenAI in a lucrative deal, as has the online forum Reddit, to Google. And given that scientific publishers probably view the alternative as their work being scraped without an agreement, “I think there will be more of these deals to come,” says Wang.
Information secrets
Some AI developers, such as the Large-scale Artificial Intelligence Network, intentionally keep their data sets open, but many firms developing generative-AI models have kept much of their training data secret, says Baack. “We have no idea what is in there,” he says. Open-source repositories such as arXiv and the scholarly database PubMed of abstracts are thought to be “very popular” sources, he says, although paywalled journal articles probably have their free-to-read abstracts scraped by big technology firms. “They are always on the hunt for that kind of stuff,” he adds.
Proving that an LLM has used any individual paper is difficult, says Yves-Alexandre de Montjoye, a computer scientist at Imperial College London. One way is to prompt the model with an unusual sentence from a text and see whether the output matches the next words in the original. If it does, that is good evidence that the paper is in the training set. But if it doesn’t, that doesn’t mean that the paper wasn’t used — not least because developers can code the LLM to filter responses to ensure they don’t match training data too closely. “It takes a lot for this to work,” he says.
Another method to check whether data are in a training set is known as membership inference attack. This relies on the idea that a model will be more confident about its output when it is seeing something that it has seen before. De Montjoye’s team has developed a version of this, called a copyright trap, for LLMs.
To set the trap, the team generates sentences that look plausible but are nonsense, and hides them in a body of work, for example as white text on a white background or in a field that’s displayed as zero width on a webpage. If an LLM is more ‘surprised’ — a measure known as its perplexity — by an unused control sentence than it is by the one hidden in the text, “that is statistical evidence that the traps were seen before”, he says.
Copyright questions
Even if it were possible to prove that an LLM has been trained on a certain text, it is not clear what happens next. Publishers maintain that, if developers use copyrighted text in training and have not sought a licence, that counts as infringement. But a counter legal argument says that LLMs do not copy anything — they harvest information content from training data, which gets broken up, and use their learning to generate new text.
Litigation might help to resolve this. In an ongoing US copyright case that could be precedent-setting, The New York Times is suing Microsoft and ChatGPT’s developer OpenAI in San Francisco, California. The newspaper accuses the firms of using its journalistic content to train their models without permission.
Many academics are happy to have their work included in LLM training data — especially if the models make them more accurate. “I personally don’t mind if I have a chatbot who writes in the style of me,” says Baack. But he acknowledges that his job is not threatened by LLM outputs in the way that those of other professions, such as artists and writers, are.
Individual scientific authors currently have little power if the publisher of their paper decides to sell access to their copyrighted works. For publicly available articles, there is no established means to apportion credit or know whether a text has been used.
Some researchers, including de Montjoye, are frustrated. “We want LLMs, but we still want something that is fair, and I think we’ve not invented what this looks like yet,” he says.
doi: https://doi.org/10.1038/d41586-024-02599-9
This story originally appeared on: Nature - Author:Elizabeth Gibney