How AI is unlocking ancient texts — and could rewrite history

From deciphering burnt Roman scrolls to reading crumbling cuneiform tablets, neural networks could give researchers more data than they’ve had in centuries
In October 2023, an e-mail pinged onto Federica Nicolardi’s phone with an image that would transform her research forever. It showed a fragment of a papyrus scroll that had been burnt in the eruption of Mount Vesuvius in ad 79. The scorched scroll was one of hundreds discovered in the remains of a luxury Roman villa in Herculeaneum, near Pompeii in Italy, in the eighteenth century. Attempts over the centuries to peel apart the scrolls’ fragile, carbonized layers left many in pieces, and scholars have been forced to accept that the rest can never be opened.
Nicolardi, a papyrologist at the University of Naples in Italy, had been enlisted in an effort to use artificial intelligence (AI) to read the unreadable. Now the latest results had arrived. The image showed a strip of papyrus packed with neat Greek lettering, glowing bright against a darker background. The writing was clearly legible, a few lines deep and stretched across nearly five columns.
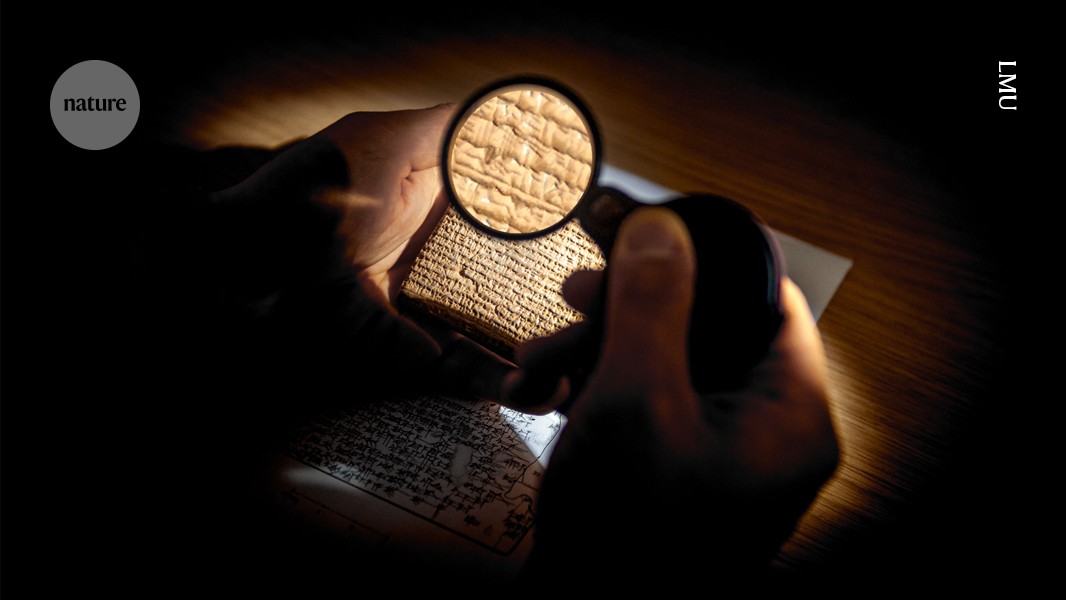
AI techniques helped to reveal the ink in this strip of charred papyrus from the Herculaneum scrolls.Credit: Vesuvius Challenge
“It was incredible,” says Nicolardi. “I thought, ‘So this is really happening.’” She knew right then that papyrology would never be the same. “In that moment, you really think ‘now I’m living something that will be a historical moment for my field.’” She was reading entire lines of a text that had been utterly inaccessible for 2,000 years.
That project, called the Vesuvius Challenge, is just one example of how sophisticated AI, which is already revolutionizing all areas of modern life, from banking to medical research, is poised to reshape how we see the ancient world. Artificial neural networks are being used to decipher ancient texts, from the classical stalwarts of Greek and Latin to China’s Oracle Bone Script, ancient divination texts written on cattle bones and turtle shells. They are making sense of archives too vast for humans to read, filling in missing and unreadable characters and decoding rare and lost languages of which hardly any traces survive.

Scrolls scorched in the eruption of Mount Vesuvius can’t be opened without damaging them.Credit: EduceLab, University of Kentucky
The results promise a flood of new texts, offering scholars more data than they have had for centuries. But that’s not all. Because AI tools can recognize more languages and store more information than any one person can know — and discover statistical patterns in texts for themselves — these technologies promise a fundamentally new way to explore ancient sources. That could transform “not only the questions we want to answer”, Nicolardi says, “but the questions we can ask”.
Reconstructing ancient texts
Computers have been used to categorize and analyse digitized texts for decades. But the current excitement comes from the use of neural networks, which comprise hierarchical layers of inter-connected nodes, and, particularly, the ‘deep’ neural networks that have multiple internal layers.
Early attempts to apply deep learning to ancient texts, in the 2010s, were based on digital photographs of texts, whether on papyri or palm leaves. Models called convolutional neural networks (CNNs) — inspired by visual neuroscience — can capture grid-like data from images. They are used for optical character recognition, but there are other applications, too: Chinese teams studying Oracle Bone Script have used such models to fill in images of eroded lettering1, analyse how oracle characters evolved over time2 and piece together broken fragments3. Meanwhile, recurrent neural networks (RNNs), designed to tackle sequences of data in which the linear order matters, began to show huge potential for searching, translating and filling in gaps in texts that have already been transcribed. They have been used, for example, to suggest missing characters in hundreds of formulaic administrative and legal texts from ancient Babylon4.
Can neural networks go beyond speeding up tedious tasks, to make connections that human specialists can’t? The first big project to show AI’s potential began life as a collaboration at the University of Oxford, UK, in 2017, where Thea Sommerschield was doing a PhD in ancient history and Yannis Assael was doing a PhD in computer science. Sommerschield was trying to decipher Greek inscriptions from Sicily and explained to Assael the challenges involved. “They’re very complex to read, they’re badly preserved, parts of them are missing,” she says. “We’re not really sure where they came from or what their dates are; there’s interesting mixes of dialects.”
Classicists interpret new sources by using their knowledge of similar existing texts. They are generally specialists on works from a particular time and place; it isn’t possible for one person to be on top of all sources potentially relevant to a new text. It was just the kind of challenge that machine-learning models could help with, suggested Assael, who is now based at Google DeepMind in London.
The researchers initially trained an RNN-based model called Pythia on tens of thousands of Greek inscriptions written between the seventh century bc and the fifth century ad. Then they showed the model texts that it hadn’t seen before, and asked it to suggest missing words or characters5.
Sommerschield, now at the University of Nottingham, UK, still remembers running the model for the first time with Assael and her supervisor, Jonathan Prag, and seeing the restoration appear character by character on the screen, something that had never been possible before.
“It was like a scene from a film,” she says. “We really felt our jaws hitting the ground.” They followed up in 2022 with a model called Ithaca, which also makes suggestions for the date and place of origin of an unknown text6. This time, the researchers took advantage of a breakthrough in machine learning called the transformer model, which captures more complex language patterns than an RNN is able to by analysing different characteristics of an input — such as characters or words — in parallel, weighting them according to the context. (Popular chatbots such as OpenAI’s ChatGPT and Anthropic’s Claude are based on transformer models.)
Sommerschield says that the team’s aim is to design tools that will help researchers to work more effectively: the neural network probes connections across a vast archive and the human brings their specialist understanding. “The human is in the centre of our design,” agrees Assael. In tests, Ithaca restored artificially produced gaps in ancient texts with 62% accuracy, compared with 25% for human experts. But experts aided by Ithaca’s suggestions had the best results of all, filling gaps with an accuracy of 72%. Ithaca also identified the geographical origins of inscriptions with 71% accuracy, and dated them to within 30 years of accepted estimates.
Ithaca is freely available online and already receives hundreds of queries a week, according to its creators. It isn’t possible to know when it has contributed to research unless the authors choose to acknowledge it, says Sommerschield, but examples reported so far include the re-dating of Athenian political decrees, and an investigation of tablets from the fourth century bc that contain questions put to the Oracle of Dodona in northwestern Greece.
An ocean of archives
South Korean researchers, meanwhile, are facing very different challenges as they tackle one of the world’s largest historical archives: detailed daily records with hundreds of thousands of articles covering the reigns of 27 Korean kings, dating from the fourteenth to the early twentieth centuries. “The amount of data is vast,” says Kyunghyun Cho, a leading machine-translation researcher at New York University in New York City. Cho usually works with modern languages, but became interested in the archives after discussing them with his father, a retired professor of Korean literature. These records are complete and their origins are known, but hardly anyone can read them. They are written in Hanja, an ancient writing system based on Chinese characters that is different from modern Chinese or Korean.

Archives of Korean kings from the Joseon dynasty are being translated and analysed by AI.Credit: National Palace Museum of Korea
A small team of government translators is working to translate the texts into modern Korean manually, but the task is likely to take decades to finish. Working with colleagues in South Korea, including JinYeong Bak at Sungkyunkwan University in Seoul, Cho trained a transformer-based network to translate the records automatically7. Not enough material has yet been translated into modern Korean to train such a model, so the team took a multilingual approach, using Hanja, translations made several decades ago into archaic Korean and the limited number of modern translations into both Korean and English. Human specialists rated the AI translations — descriptions of events such as state visits, punishment of traitors and musical concerts — as significantly more accurate and readable than the archaic ones, and in some cases better than the modern translation8.
At the other end of the scale, researchers are using neural networks to tackle ancient languages for which only a small amount of text survives. Transformer models can’t always be used in these cases, because they need large amounts of training material. For example, Katerina Papavassileiou at the University of Patras, Greece, and her colleagues used an RNN to restore missing text from a series of 1,100 Mycenaean tablets from Knossos, Crete, containing accounts of sheep herds written in a script called Linear B in the second millennium bc9. In tests with artificially produced gaps, the model’s top ten predictions included the correct answer 72% of the time, and in real-world cases it often matched the suggestions of human specialists. To improve the results further, Papavassileiou hopes to add in visual data, such as traces of incomplete letters, rather than just relying on the transliterated text. She is also investigating ‘transfer learning’, in which the model applies lessons learnt from one series of tablets to another10.
Papavassilieou hopes to one day use models trained on Linear B to tackle Linear A, a script used by the Minoan civilization that shares many symbols with Linear B but has never been deciphered.

Clay tablets from the Minoan civilization (fifteenth century bc) with the Linear A script, which has never been deciphered. These were found in Archanes, Crete.Credit: DeAgostini/Getty
Deciphering the unreadable
Perhaps the ultimate proof of AI’s power to solve monumental challenges is the success of researchers studying the Herculaneum scrolls. “I think they are doing some of the most amazing work out there,” says Assael. Computer scientist Brent Seales and his colleagues at the University of Kentucky in Lexington, aided by Vesuvius Challenge participants, are tackling the seemingly impossible task of reading text that can’t be seen at all.
Reading the Herculaneum scrolls involves overcoming two big problems. First, the fragile scrolls can’t be unwound. To see inside them, Seales spent years developing ‘virtual unwrapping’ technology, which involves taking high-resolution computed tomography (CT) scans of a scroll’s internal structure, painstakingly mapping by hand the surfaces visible in each frame of the cross section, then using algorithms to unroll the surfaces into a flat image. In 2015, the researchers used this technique to read complete text from inside a charred, unopenable scroll from En-Gedi in Israel, dated to around the third century ad, which turned out to be from the biblical Book of Leviticus11.
The En-Gedi scroll has five wraps; the Herculaneum scrolls each contain hundreds of turns, as thin as silk. So to capture extremely high resolution CT data, the team transported several of the scrolls to the Diamond Light Source particle accelerator near Oxford. But whereas the ink of the En-Gedi scroll and other later works tends to contain iron, which glows brightly in CT scans, the scribes of Herculaneum used carbon-based ink, invisible in scans because it has the same density as the papyrus it sits on. Seales and his team realized that although they couldn’t see the ink directly, they might be able to detect its shape. If there was a subtle difference in the surface texture of bare papyrus fibres compared with ink-coated ones, perhaps they could train a neural network to spot the difference.

A piece of a Herculaneum scroll at the UK Diamond Light Source synchrotron in 2019, where it was scanned.Credit: Geoff Caddick/AFP/Getty
It was too much work for Seales’ small team, so they teamed up in March 2023 with Silicon Valley entrepreneur Nat Friedman to launch the Vesuvius Challenge, which offered big cash prizes. Seales and his colleagues released flattened images of scroll surfaces and asked the contestants to train neural networks to find the ink. More than 1,000 teams competed, with hundreds of people discussing progress on the contest’s Discord channel every day. A grand prize was awarded in February 2024: computer-science students Youssef Nader, Luke Farritor and Julian Schilliger together received US$700,000 for producing 16 columns of clearly readable text.
The winning team used a TimeSformer, a more recent variant of the transformer model, usually used for videos, that attends to spatial and time dimensions separately. The Vesuvius team used it to separate the depth dimension of the papyrus from the appearance of its surface. Nicolardi and her colleagues subsequently identified the revealed text as from a previously unknown work of Greek philosophy on music, pleasure and sensation, possibly by the Epicurean philosopher Philodemus. To work on it was “magical”, she says.

Almost 16 columns of Greek writing were revealed inside this charred Herculaneum scroll, by the February 2024 winners of the Vesuvius Challenge.Credit: Vesuvius Challenge
Since then, contestants have been working to improve their ink-detection algorithms, with help from the papyrologists. Meanwhile, Seales’ team is scanning more scrolls, and hopes that machine learning can speed up the virtual-unwrapping step. That’s the bottleneck currently limiting the data that contestants have to work with, he says. He’s optimistic that AI-driven unwrapping will be available in time for someone to win the 2024 Grand Prize, of $200,000, for reading 90% of four scrolls. “Once you automate it, you can basically go to scale,” says Seales about the unwrapping. “We’re kind of on the cusp of that.”
In fact, Seales wants to read the whole library. There are hundreds of unopened scrolls from Herculaneum held in collections — mostly in Naples, but also in Paris, London and Oxford. “That’s going to be more text for the papyrologists that’s new from the ancient world than they’ve seen in a century,” he says.

An animation shows how ink-detection models were used to read text from the Herculaneum scrolls.Credit: Vesuvius Challenge
The method also opens up other inaccessible sources, what Seales calls “the invisible library”. These include texts hidden inside medieval book bindings or ancient Egyptian mummy wrappings, for which “it’s here, and we hold the physical object, but we can’t read the writing”. Already, the team has captured data from an unopened Egyptian scroll held in the Smithsonian museum in Washington DC, and is in discussions to analyse papyri from Petra, Jordan, that were burnt in a fire in the seventh century ad.
What’s more, some archaeologists think that most of the Herculaneum villa’s library is still underground. If that were ever excavated, it could yield thousands more scrolls. Reading all of them would be “the biggest discovery in the history of humankind, from the ancient world”, says Seales. “Now, we have the technology.”
A flood of information
Enjoying our latest content?
Login or create an account to continue
- Access the most recent journalism from Nature's award-winning team
- Explore the latest features & opinion covering groundbreaking research
or
Sign in or create an accountNature 637, 14-17 (2025)
doi: https://doi.org/10.1038/d41586-024-04161-z
This story originally appeared on: Nature - Author:Jo Marchant