AI watermarking must be watertight to be effective But the technology’s robustness remains a challenge

Scientists are closing in on a tool that can reliably identify AI-generated text without affecting the user’s experience
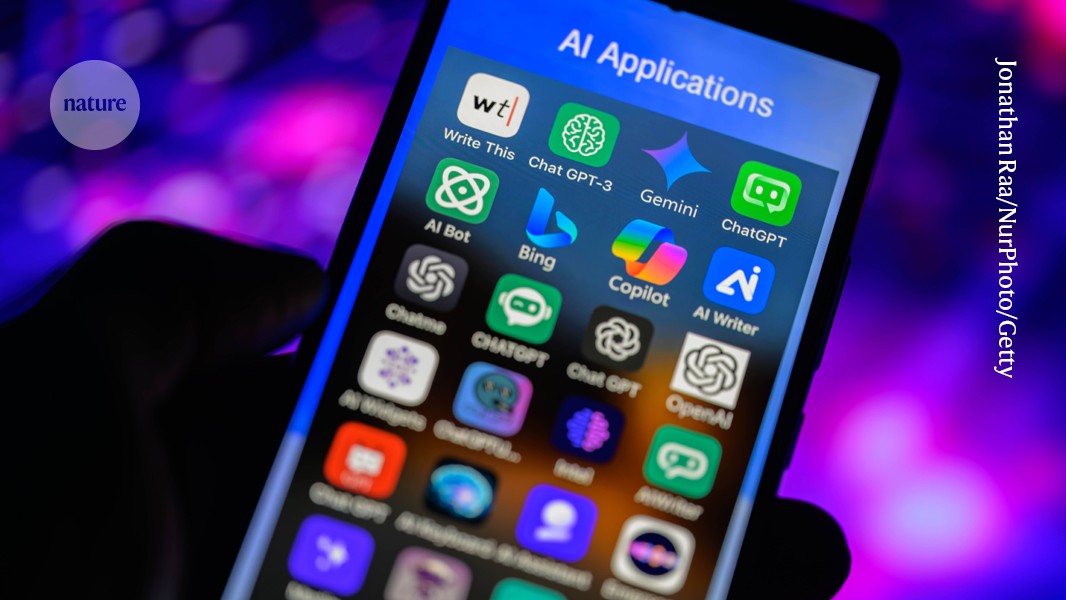
Watermarking could soon be coming to an AI chatbot near you.Credit: Jonathan Raa/NurPhoto/Getty
Seldom has a tool or technology erupted so suddenly from the research world and into the public consciousness — and widespread use — as generative artificial intelligence (AI). The ability of large language models (LLMs) to create text and images almost indistinguishable from those created by humans is disrupting, if not revolutionizing, countless fields of human activity. Yet the potential for misuse is already manifest, from academic plagiarism to the mass generation of misinformation. The fear is that AI is developing so rapidly that, without guard rails, it could soon be too late to ensure accuracy and reduce harm1.
This week, Sumanth Dathathri at DeepMind, Google’s AI research lab in London, and his colleagues report their test of a new approach to ‘watermarking’ AI-generated text by embedding a ‘statistical signature’, a form of digital identifier, that can be used to certify the text’s origin2. The word watermark comes from the era of paper and print, and describes a variation in paper thickness, not usually immediately obvious to the naked eye, that does not change the printed text. A watermark in digitally generated text or images should be similarly invisible to the user — but immediately evident to specialized software.
Dathathri and his colleagues’ work represents an important milestone for digital-text watermarking. But there is still some way to go before companies and regulators will be able to confidently state whether a piece of text is the product of a human or a machine. Given the imperatives to reduce harm from AI, more researchers need to step up to ensure that watermarking technology fulfils its promise.
The authors’ approach to watermarking LLM outputs is not new. A version of it is also being tested by OpenAI, the company in San Francisco, California, behind ChatGPT. But there is limited literature on how the technology works and its strengths and limitations. One of the most important contributions came in 2022, when Scott Aaronson, a computer scientist at the University of Texas at Austin, described, in a much-discussed talk, how watermarking can be achieved. Others have also made valuable contributions — among them John Kirchenbauer and his colleagues at the University of Maryland in College Park, who published a watermark-detection algorithm last year3.
The DeepMind team has gone further, demonstrating that watermarking can be achieved on a large scale. The researchers incorporated a technology that they call SynthID-Text into Google’s AI-powered chatbot, Gemini. In a live experiment involving nearly 20 million Gemini users who put queries to the chatbot, people didn’t notice a diminution in quality in watermarked responses compared with non-watermarked responses. This is important, because users are unlikely to accept watermarked content if they see it as inferior to non-watermarked text.
However, it is still comparatively easy for a determined individual to remove a watermark and make AI-generated text look as if it was written by a person. This is because the watermarking process used in DeepMind’s experiment works by subtly altering the way in which an LLM statistically selects its ‘tokens’ — how, in the face of a given user prompt, it draws from its huge training set of billions of words from articles, books and other sources to string together a plausible-sounding response. This alteration can be spotted by an analysing algorithm. But there are ways in which the signal can be removed — by paraphrasing or translating the LLM’s output, for example, or asking another LLM to rewrite it. And a watermark once removed is not really a watermark.
Getting watermarking right matters because authorities are limbering up to regulate AI in a way that limits the harm it could cause. Watermarking is seen as a linchpin technology. Last October, US President Joe Biden instructed the National Institute of Standards and Technology (NIST), based in Gaithersburg, Maryland, to set rigorous safety-testing standards for AI systems before they are released for public use. NIST is seeking public comments on its plans to reduce the risks of harm from AI, including the use of watermarking, which it says will need to be robust. There is no firm date yet on when plans will be finalized.
In contrast to the United States, the European Union has adopted a legislative approach, with the passage in March of the EU Artificial Intelligence Act, and the establishment of an AI Office to enforce it. China’s government has already introduced mandatory watermarking, and the state of California is looking to do the same.
However, even if the technical hurdles can be overcome, watermarking will only be truly useful if it is acceptable to companies and users. Although regulation is likely, to some extent, to force companies to take action in the next few years, whether users will trust watermarking and similar technologies is another matter.
There is an urgent need for improved technological capabilities to combat the misuse of generative AI, and a need to understand the way people interact with these tools — how malicious actors use AI, whether users trust watermarking and what a trustworthy information environment looks like in the realm of generative AI. These are all questions that researchers need to study.
In a welcome move, DeepMind has made the model and underlying code for SynthID-Text free for anyone to use. The work is an important step forwards, but the technique itself is in its infancy. We need it to grow up fast.
Nature 634, 753 (2024)
doi: https://doi.org/10.1038/d41586-024-03418-x
This story originally appeared on: Nature - Author:furtherReadingSection