Why is it so hard to rewrite a genome? But the hidden complexity of biological systems continues to surprise them

Synthetic biologists have the know-how and ambition to retool whole genomes
When Patrick Yizhi Cai reflects on the state of synthetic genomics, he recalls the Big DNA Contest. Launched in 2004, the competition challenged synthetic biologists to design a novel, functional 40,000-base-pair DNA sequence that the contest sponsor, US DNA-synthesis firm Blue Heron Biotech (now Eurofins Genomics Blue Heron) would manufacture for free.
It was no small prize: at the time, producing this modest slab of DNA — less than 1% the length of the Escherichia coli genome — would have cost roughly US$250,000. The company’s aim was to energize the then-nascent field of synthetic biology. “In the end, zero applications were received,” says Cai, a synthetic biologist at the University of Manchester, UK. “That just tells you that even if you could make synthetic DNA for free, nobody really had enough imagination 20 years ago.”
Today, steady progress in genomics and computational biology — not to mention DNA synthesis and assembly — have yielded multiple examples of what an ambitious, imaginative genome-writing effort can achieve. The synthetic bacterial strain JCVI-syn3A, developed at the J. Craig Venter Institute (JCVI) in La Jolla, California, is a streamlined version of Mycoplasma mycoides that survives and replicates despite having had several hundred non-essential genes stripped away1. Various groups are engineering E. coli strains in which the genetic code has been altered to enable production of proteins containing amino acids beyond the 20 typically observed in nature. And last year, the multinational Synthetic Yeast Genome Project (Sc2.0) completed construction of heavily engineered versions of every chromosome in the eukaryotic budding yeast, Saccharomyces cerevisiae — comprising some 12 million base pairs in all.
These efforts have been invaluable learning experiences, says Akos Nyerges, a synthetic-genomics researcher involved with the E. coli rewriting effort in George Church’s lab at Harvard Medical School in Boston, Massachusetts. “You can mimic and test evolutionary steps which otherwise would have taken billions of years to evolve — or wouldn’t have evolved ever,” he says. But they have also laid bare how much we still don’t understand about the fundamental language of the genome. Every genome-rewriting program so far has grappled with substantial and unexpected challenges, and the era of made-to-order genomes remains out of reach. When it comes to heavily modified genomes, says Nyerges, “we underestimated how complex biology is”.
Back to basics
Most synthetic-genome projects are ‘top-down’ efforts that take a naturally occurring organism and pare away or redesign its DNA. That provides a valuable initial framework relative to ‘bottom-up’ approaches, in which the goal is to build a working genome from scratch. After all, explains Farren Isaacs, a genome engineer at Yale University in New Haven, Connecticut, when it comes to tinkering with genomes, the margin for error is surprisingly slim. “If you create an error in an essential gene, you’re going to wipe the organism out.”
A key goal of the JCVI and Sc2.0 projects was to determine which genes are truly essential — a characteristic that is surprisingly hard to predict. John Glass, leader of the JCVI’s synthetic-biology programme, says that when he and his team published2 their 2016 report on their first minimal cell, nearly one-third of the cell’s remaining genes (149 of 473) had no known function. “I’d say it’s 78 now,” he adds.
To determine which genes were necessary, both projects used random mutagenesis — basically, introducing untargeted perturbations throughout the genome and asking which ones the cells could tolerate and which ones severely undermined cellular viability.
But essentiality is a slippery concept, particularly given that most genomes contain redundancies and ‘fail-safe’ mechanisms to minimize the impact of individual mutations. Glass and his colleagues encountered dozens of instances in which mutagenesis revealed pairs of seemingly dissimilar genes that unexpectedly performed overlapping functions. As a result, there is no single minimal genome, he explains. “You take away one [gene], and with each choice, you’re going down a different road to a slightly different minimal cell.” Furthermore, many bacterial genes have multiple jobs, making it difficult to recognize which is the essential function. Glass cites the example of enolase, an enzyme with a well-known role in carbohydrate metabolism that also, it turns out, helps to degrade unwanted RNA.
Increasingly sophisticated computational ‘whole-cell models’ could help to remove some of the guesswork from future genome-trimming efforts. In 2020, mathematician Lucia Marucci and synthetic biologist Claire Grierson, both at the University of Bristol, UK, led an effort to simulate genome-reduction strategies in a whole-cell model of Mycoplasma genitalium — a close relative of the microorganism edited by the JCVI3. Their analysis, which used elaborate models of cellular processes and their interactions, suggested two redesigns with distinct sets of genes deleted, each yielding genomes that were roughly 40% smaller than the natural M. genitalium genome.
More recently, Marucci and Grierson have begun working with sophisticated whole-cell models of E. coli. As described in a 2024 preprint4, their current efforts combine mechanistic models with machine learning to predict the consequences of genome manipulation across a broad range of biological functions. These are described by thousands of interlinked equations, yielding blueprints for bacteria that have 40% fewer genes than wild-type E. coli. “We now have a bunch of minimized reduced genomes that we want to test in the laboratory,” Marucci says.
Find and replace
Rather than making abridged editions of the genome, other groups have set out to subtly reword the genetic text — encountering an entirely different set of challenges.
Protein-coding sequences are built of nucleotide triplets known as codons. With 61 possible codons for the 20 naturally occurring amino acids as well as 3 ‘stop’ codons that terminate protein synthesis, there is considerable redundancy in the resulting code. Various teams have shown that, by comprehensively converting each instance of a given codon to one of its ‘synonyms’, one can repurpose that codon. This month, for example, Isaacs and his colleagues described an E. coli strain called Ochre in which two stop codons were reassigned to direct the incorporation of the non-natural amino acids para-acetyl-l-phenylalanine and Nε-Boc-l-lysine5. These amino acids have chemical properties and functions that don’t exist in nature, but recoding can also serve as a ‘firewall’ that prevents the interaction and exchange of genetic material with other organisms in natural environments.
Such work might sound straightforward — simply substituting one codon for another — but genome recoding requires much planning and effort. After researchers have found all instances of the codon they wish to eliminate, they must then work out how to replace it without disrupting the affected genes or regulatory machinery. Bacterial genes often contain regulatory sequences in the protein-coding sequence, Nyerges points out, and a gene on one DNA strand can overlap with a gene on the opposite strand. Seemingly minor changes can thus have major, unexpected consequences.
Nyerges, Church and their colleagues are grappling with this challenge at an unprecedented scale as they finalize a heavily recoded variant of E. coli that uses only 57 of the 61 naturally occurring amino-acid codons6. This effort has entailed more than 73,000 changes to the strain’s 4-megabase genome, which inevitably creates unintended effects. “Some things will happen readily with no impact on growth or fitness, while others have a striking impact,” says Nyerges. Some changes disabled existing regulatory elements or unwittingly created new one; others established new protein-coding sequences. “And we’re only learning about this as we go.”
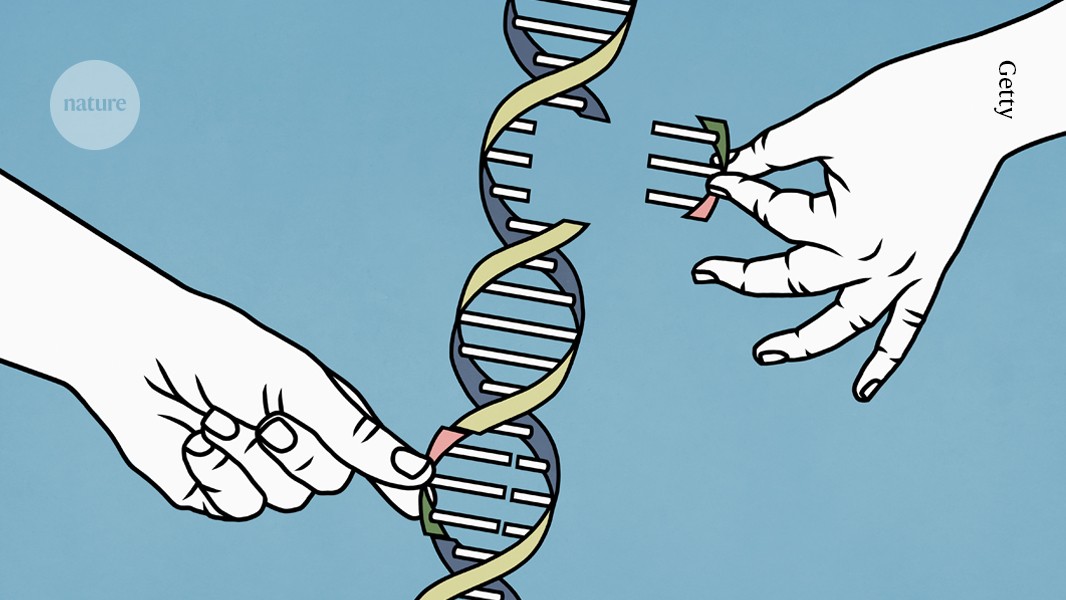
Computer-generated models of the synthetic minimal cell created by researchers at the J. Craig Venter Institute in La Jolla, California.Credit: Laboratory of Zaida Luthey-Schulten
Sorting out these issues is a substantial undertaking in its own right. For example, throughout the recoding process for their Ochre strain, Isaacs and his team used extensive ‘multi-omic’ analyses to characterize the bacterium. “We collected metabolic profiling data under different [culture] conditions,” he says. “We also collected proteomics data comparing the recoded cell to a few different progenitors, including wild-type cells.” In this way, they systematically tweaked the genome until the cells were able to grow under standard culture conditions at roughly the same rate as unmodified bacteria — a non-trivial result, given that genome recoding often impairs growth. Nyerges and his colleagues likewise turned to multi-omics to troubleshoot their 57-codon genome. They also used an experimental strategy that spurs rapid evolution of bacteria in culture, to promote the selection of genome mutations that improve fitness.
Algorithmic tools are also helping researchers to model and predict the outcomes of some genome-rewriting experiments in advance. For instance, synthetic biologist Howard Salis’s team at Pennsylvania State University in University Park uses quantitative data from high-throughput screens of both genetically modified cells and strands of synthetic DNA to develop algorithms that can define, characterize and even design sequences that govern processes such as transcription and translation. “A typical paper for us nowadays is anywhere from 10,000 to 100,000 different defined, designed experiments,” says Salis. The results are used to extract testable physical principles that allow the algorithms to predict, for example, how changes to a gene’s promoter sequence alter downstream expression.
“You can ground-truth everything,” says Salis. “And we can combine our existing models to design the next experiments to understand the remaining misunderstood stuff.” Indeed, the Church lab has used several of Salis’s tools to design its 57-codon microbe. Nyerges says such algorithms have been a substantial asset — albeit not enough to prevent considerable troubleshooting. “Even very tiny changes can cumulatively lead to significant fitness problems once you add up thousands of genes in a genome,” he says.
Brewing progress in eukaryotes
Enjoying our latest content?
Login or create an account to continue
- Access the most recent journalism from Nature's award-winning team
- Explore the latest features & opinion covering groundbreaking research
or
Sign in or create an accountNature 638, 848-850 (2025)
doi: https://doi.org/10.1038/d41586-025-00462-z
This story originally appeared on: Nature - Author:Michael Eisenstein