AI protein-prediction tool AlphaFold3 is now open source

The code underlying the Nobel-prize-winning tool for modelling protein structures can now be downloaded by academics
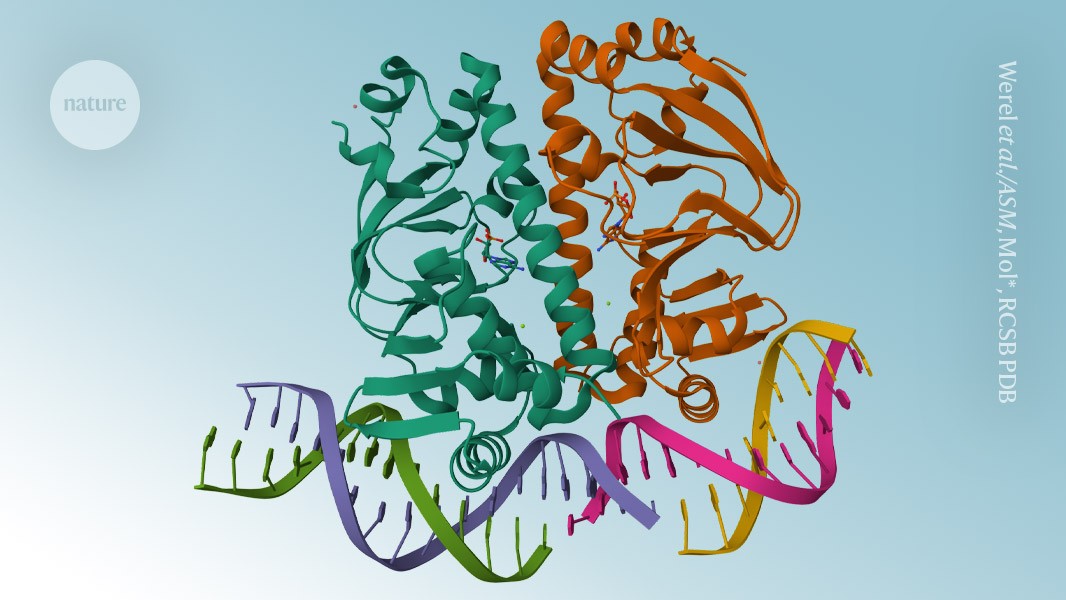
AlphaFold3 can predict the structures of proteins as they interact with DNA.Credit: Werel et al./American Society for Microbiology, Mol*, RCSB PDB
AlphaFold3 is open at last. Six months after Google DeepMind controversially withheld code from a paper describing the protein-structure prediction model, scientists can now download the software code and use the artificial intelligence (AI) tool for non-commercial applications, the London-based company announced on 11 November.
“We’re very excited to see what people do with this,” says John Jumper, who leads the AlphaFold team at DeepMind and last month, along with CEO Demis Hassabis, won a share of the 2024 Chemistry Nobel Prize for their work on the AI tool.
AlphaFold3, unlike its predecessors, is capable of modelling proteins in concert with other molecules. But instead of releasing its underlying code — as was done with AlphaFold2 — DeepMind provided access via a web server that restricted the number and types of predictions scientists could make.
Crucially, the AlphaFold3 server prevented scientists from predicting how proteins behave in the presence of potential drugs. But now, DeepMind’s decision to release the code means academic scientists can predict such interactions by running the model themselves.
The company initially said that making AlphaFold3 available only through a web server struck the right balance between enabling access for research and protecting commercial ambitions. Isomorphic Labs, a DeepMind spinoff company in London, is applying AlphaFold3 to drug discovery.
But the publication of AlphaFold3 without its code or model weights — parameters obtained by training the software on protein structures and other data — drew criticism from scientists, who said the move undermined reproducibility. DeepMind swiftly reversed course and said it would make an open-source version of the tool available within half a year.
Anyone can now download the AlphaFold3 software code and use it non-commercially. But for now, only scientists with an academic affiliation can access the training weights on request.
Accessible versions
DeepMind has got competition: over the past few months, several companies have unveiled open-source protein structure prediction tools based on AlphaFold3, relying on specifications described in the original paper known as pseudocode.
Two Chinese companies — technology giant Baidu and TikTok developer ByteDance — have rolled out their own AlphaFold3 inspired models, as has a start-up in San Francisco, California, called Chai Discovery.
A key limitation of these models is that, like AlphaFold3, none is licensed for commercial applications such as drug discovery, says Mohammed AlQuraishi, a computational biologist at Columbia University in New York City. However, Chai Discovery’s model, Chai-1, can be used via a web server for such work, says Jack Dent, a co-founder of the company.
Another firm, San Francisco-based Ligo Biosciences, has released a restriction-free version of AlphaFold3. But it doesn’t yet have the full suite of capabilities, including the capacity to model drugs and molecules other than proteins.
Other teams are working on versions of AlphaFold3 that don’t come with such limits: AlQuraishi hopes to have a fully open-source model called OpenFold3 available by the end of the year. This would enable drug companies to retrain their own versions of the model using proprietary data, such as the structures of proteins bound to different drugs, potentially improving performance.
Openness matters
The last year has seen a flood of new biological AI models released by companies with varying approaches to openness. Anthony Gitter, a computational biologist at the University of Wisconsin-Madison, has no problem with for-profit companies joining his field — so long as they play by the same rules as other scientists when they share their work in journals and preprint servers.
If DeepMind makes claims about AlphaFold3 in a scientific publication, “I and others expect them to also share information about how predictions were made and put the AI models and code out in a way that we can inspect,” Gitter adds. “My group's not going to build on and use the tools that we can't inspect.”
The fact that several AlphaFold3 replications have already emerged shows that model was reproducible, even without open-source code, says Pushmeet Kohli, DeepMind’s head of AI for science. He adds that in future he would like to see more discussion about the publishing norms in a field increasingly populated by both academic and corporate researchers.
The open-source nature of AlphaFold2 led to a flood of innovation from other scientists. For instance, the winners of a recent protein design contest used the AI tool to design new proteins capable of binding a cancer target. Jumper’s favourite recent AlphaFold2 hack was from a team that used the tool to identify a key protein that helps sperm attach to egg cells.
Jumper can’t wait for such surprises to emerge after sharing AlphaFold3 — even if they don’t always bear fruit. “People will use it in weird ways,” he predicts. “Sometimes it will fail and sometimes it will succeed.”
doi: https://doi.org/10.1038/d41586-024-03708-4
This story originally appeared on: Nature - Author:Ewen Callaway