Five structural-biology questions that still challenge AI But some problems remain out of reach — for now

Tools such as Rosetta and AlphaFold have redefined the protein-engineering landscape
Alena Khmelinskaia wants designing bespoke proteins to be as simple as ordering a meal. Picture a vending machine, she says, which any researcher could use to specify their desired protein’s function, size, location, partners and other characteristics. “Ideally, you would get the perfect design that can accomplish all these things together,” says Khmelinskaia, a biophysical chemist at Ludwig Maximilian University in Munich, Germany.
For the moment, that is just a dream. But advances in computational protein design and machine learning are bringing it closer to reality than ever.
Until a few years ago, researchers altered proteins by cloning them into bacteria or yeast, and coaxing the microorganisms to mutate until they produced the desired product. Scientists could also design a protein manually by deliberately altering its amino-acid sequence, but that’s a laborious process that could cause it to fold incorrectly or prevent the cell from producing it at all.
Machine-learning algorithms have changed the game entirely. Researchers can generate new protein structures on their laptops using tools driven by artificial intelligence (AI), such as RFdiffusion and Chroma, which were trained on hundreds of thousands of structures in the Protein Data Bank (PDB). They can identify a sequence to match that structure using algorithms such as ProteinMPNN. RoseTTAFold and AlphaFold, which calculate structures from a sequence, can predict whether the new protein is likely fold correctly. Only then do researchers need to synthesize the physical protein and test whether it works as predicted.
In many cases, it does. “Once people see the experimental data, they get that this thing can work,” Khmelinskaia says of AI protein design. “There’s excitement for what is possible.” This year’s Nobel chemistry prize committee agrees: AlphaFold and other programs that predict or design protein structures won their developers the 2024 prize. “That we can now predict protein structures and design our own proteins confers the greatest benefit to humankind,” the announcement read.
Still, the greatest benefits could be yet to come. Nature spoke to specialists about the biggest challenges facing protein design and what it will take to overcome them. Here’s what they said.
Building reliable binders
One of the early challenges for protein designers was to predict how proteins bind to one another — a major goal for the pharmaceutical industry, because ‘binders’ for a given protein could serve as drugs that activate or inhibit disease pathways. Generative AI programs such as RFdiffusion and AlphaProteo have made this task straightforward, says David Baker, a pioneer of computational protein design and 2024 Nobel chemistry laureate at the University of Washington in Seattle, whose team developed RFdiffusion and other protein-design tools. “If you want to target some cancer protein, for example, and you’d like a binder to it, the methods we’ve developed will generally give you a solution to that problem,” he says.
Some proteins, such as the transmembrane molecules that stud the surfaces of immune cells, remain tough to crack. But for most proteins, generative AI software can generate binders that wrap precisely around their target, like a hand. For instance, in 2023, Baker and his colleagues used RFdiffusion to create sensor proteins that light up when they attach to specific peptide hormones1.
Protein–protein binding algorithms have been successful because their language is simple: all natural proteins are made of the same 20 amino acids. And with hundreds of thousands of structures and protein–protein interactions available in the PDB, “that’s kind of like an ideal case for machine learning”, says computer scientist John Ingraham at Generate Biomedicines, a company in Somerville, Massachusetts, that uses AI to design therapeutics. Teams such as his have been using AI tools to design large libraries of simple binding proteins, in the hope of applying them to research problems.
But binders become less reliable the fewer data the AI has to train on, as is the case for proteins intended to bind to drugs and other small molecules. Many pharmaceutical companies have their own databases of small-molecule structures and how they interact with proteins, but these are closely held secrets. The public data that exist are not always well annotated, and the structures that are available tend to represent just a few molecular classes, says Jue Wang, a computational biologist at Google DeepMind in London. “With a model trained on that, you might not necessarily learn good general rules about chemistry,” he says.
Earlier this year, DeepMind released AlphaFold3, the software’s latest iteration, which predicts how binding to small molecules affects a protein’s shape. “For the interactions of proteins with other molecule types, we see at least a 50% improvement compared with existing prediction methods, and for some important categories of interaction we have doubled prediction accuracy,” the company says.
But the challenge isn’t completely solved, Baker says. For instance, just because something binds well doesn’t mean it will work as intended. A binder protein can activate its target or block it, but programs such as AlphaFold cannot necessarily tell the difference, Khmelinskaia says. (Some algorithms do incorporate function, she notes, including ESM3. Developed by a company called EvolutionaryScale in New York City, that software was trained on 2.7 billion protein sequences, structures and functions.)
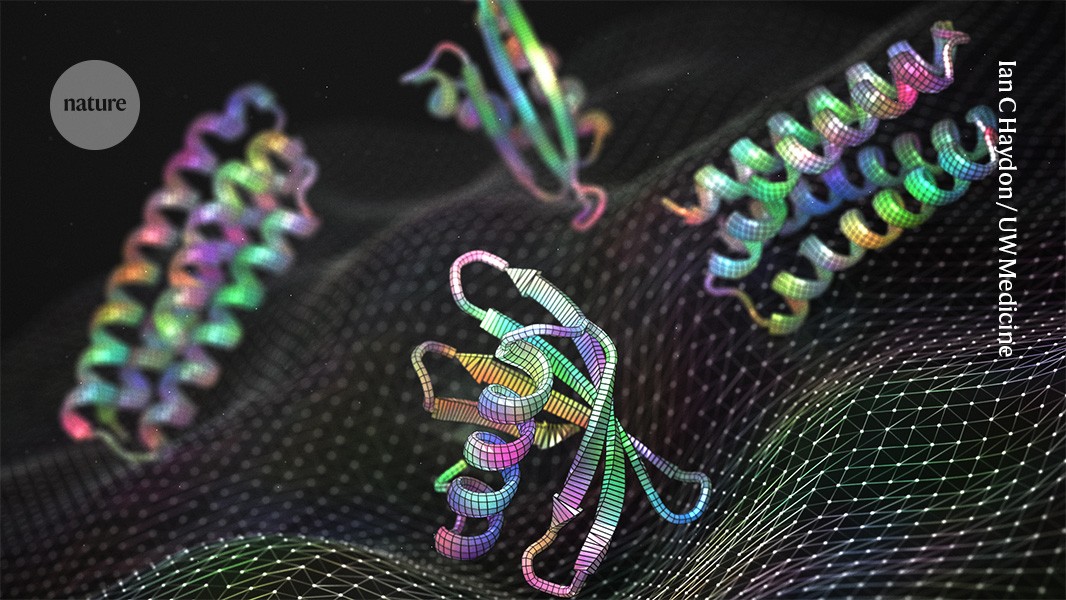
Alena Khmelinskaia is developing hollow nanoparticles with the help of machine learning.Credit: Bhoomika Basu Mallik
Generative AI systems have other limitations, including a tendency to ‘hallucinate’ protein structures that cannot exist in nature. The AI is “always trying to please”, says Mohammed AlQuraishi, a computational biologist at Columbia University in New York City. “It never, ever says, ‘no, this is not doable’.”
A better understanding of biophysics might help, Ingraham says, but so would more and better data on how proteins bind to molecules. His company is attacking the problem through brute force, using as much data on protein interactions and functions as possible and combining it with high-throughput data on designs generated by their model. “We’re trying to find general solutions,” he says, “then just leverage as much protein information as we can.”
New catalysts
Scientists have high hopes that computational tools will lead to enzymes with entirely new functions: catalysts that can scrub carbon dioxide from the atmosphere, for instance, or enzymes that efficiently break down environmental plastics. The logical place to start is with natural enzymes that perform similar functions. An enzyme that breaks hydrogen–silicon bonds, for instance, might form the scaffold for an artificial enzyme that breaks carbon–silicon bonds.
But similar protein shapes don’t necessarily equate to similar functions, and enzymes that look nothing alike can carry out identical tasks. Working out those connections — and how to recreate functions — is a major challenge in protein design, AlQuraishi says. “We don’t speak function, we speak structure.”
Moreover, natural enzymes are not necessarily ideal starting points for a new intended activity. Debora Marks, a systems biologist at Harvard Medical School in Boston, Massachusetts, likens repurposing enzymes to building a modern road system atop a city’s existing, antiquated layout. “If you could start again, you wouldn’t necessarily do it like that,” she says.
That said, the biophysics of natural enzymes can inform de novo designs, Marks says: “Nature has done billions of evolutionary experiments for you.” Typically, researchers determine which parts of an enzyme are important by analysing how similar they are across species. Evolutionarily conserved sequences often have similar structures, whereas dissimilar ones might just be junk that slows an enzyme down.
But it’s not always immediately apparent which parts are important, Ingraham says. A seemingly useless amino-acid chain on the side of an enzyme, for instance, might affect how tightly a protein can bind to other molecules or its ability to flip between conformational states.
Some researchers are developing methods for finding those useful parts. In an August preprint, Baker and his colleagues used RFdiffusion to create a set of enzymes known as hydrolases, which use water to break chemical bonds through a multistep process2. Using machine learning, the researchers analysed which parts, or motifs, of the enzymes were active at each step. They then copied these motifs and asked RFdiffusion to build entirely new proteins around them. When the researchers tested 20 of the designs, they found that two of them were able to hydrolyse their substrates in a new way. “That had been a goal for a long time, and that’s been solved,” Wang says.
Still, moving active sites into new protein environments can be tricky, warns Martin Steinegger, a computational biologist at Seoul National University. Without the rest of its protein to stabilize the structure or perform functions that researchers haven’t yet identified, an isolated motif might bind to its target and never let go. Proteins, Steinegger explains, are not static objects, but dynamic. “Whenever dynamics comes in, we are just not really great in modelling this.”
Conformational changes
Proteins generally don’t have just one shape; they open, close, twist and flex. These conformations change depending on factors such as temperature, pH, the chemical environment, and whether they’re bound to other molecules.
Yet, when researchers attempt to solve the structure of a protein experimentally, they often end up seeing only the most stable conformation, which isn’t necessarily the form the protein takes when it’s active. “We take these snapshots of them, but they’re wiggly,” says Kevin Yang, a machine-learning scientist at Microsoft Research in Cambridge, Massachusetts. To truly understand how a protein works, he says, researchers need to know the whole range of its potential movements and conformations — alternative forms that aren’t necessarily catalogued in the PDB.
Calculating all the ways in which proteins might move is astronomically difficult, even for a supercomputer. A protein with 100 amino acids — small by protein standards — could assume at least 3100 possible conformations, says Tanja Kortemme, a bioengineer at the University of California, San Francisco. “Our understanding of physics is pretty good, but incorporating this is limited by the number of possibilities we need to compute.”

Protein-design pioneer David Baker on the day it was announced that he would share in the 2024 chemistry Nobel prize.Credit: Zach Garcia / UW Medicine
Machine learning can help to narrow them down, and Microsoft and other companies are developing ways to speed up the calculations needed to find a protein’s conformation. But AI models are limited by a lack of good training data, Wang says: “Ground truth actually generally doesn’t exist, so how do you know you’ve even gotten the right answer?”
Kortemme says the field is chipping away at this problem by designing large libraries of proteins — both natural and synthetic — and mutating them to reveal their dynamics. For instance, she, Baker and others are working on proteins that can be manually switched between two conformations by adding certain binding partners3. Such designer proteins could not only help to train AI models but also serve as building blocks for more-complex molecular machines, such as enzymes that convert chemical energy to mechanical energy to do cellular work.
Other teams have developed algorithms (such as AF-Cluster) that inject a degree of randomness into their predictions to explore alternative conformations. But whether those approaches will be applicable across protein classes remains unclear, Steinegger says.
Complex creations
Enzymes aren’t the only protein class that researchers care about. New proteins could also prove useful as building blocks, for instance by self-assembling into structures that carry cargo into cells, generate physical force, or unfold misfolded proteins in disorders such as Alzheimer’s.
Computational design of these complex structures is already making an impact. In 2022 and 2023, respectively, South Korea and the United Kingdom approved emergency use of a COVID-19 vaccine that was the first medical product made from computationally designed proteins. Known as SKYCovione, the vaccine is a nanoparticle with two protein components that spark an immune response against the spike protein of the virus SARS-CoV-2. In clinical trials, SKYCovione generated three times the level of antibodies as did a commercial vaccine, and its success, Khmelinskaia says, shows that computational protein design is ready for the real world. “Now it’s really possible to start targeting a lot of interesting pathways that previously were not really possible,” she says.
Khmelinskaia’s laboratory is using machine-learning algorithms to develop hollow nanoparticles that could, among other things, carry drugs or toxins into cells or sequester unwanted molecules. That requires understanding the designed proteins’ conformational dynamics, she says, in that the particle and its payload need to be able to pass through the cell’s membrane and then open (or close).
But that’s just one function. With a more complex structure such as the bacterial flagellum, machine learning can only do so much — there just aren’t enough well-understood examples to work from. “If we had 100,000 or a million different molecular machines, maybe we could train a generative AI method to generate machines from scratch, but there aren’t,” Baker says.
That means that human researchers need to think about the components that make up a molecular machine — a motor, for instance, or a protein that ‘walks’ along another protein — and use design tools to create those building blocks one by one. Such components might include molecular switches, wheels and axles, or ‘logic gate’ systems that only function under certain conditions. “You don’t need to reinvent the wheel every time you make a complex machine,” explains Kortemme. Her lab is designing cell-signalling molecules that could be incorporated into synthetic signal-transduction cascades.
And it is in the clever recombination of these parts that human ingenuity will come to the fore, Wang says. “We’re starting to create the screws and bolts and levers and pulleys of proteins,” he says. “But what are you going to use that pulley for? That’s the most interesting and the most challenging aspect.”
Learning from mistakes
Khmelinskaia’s vending-machine vision notwithstanding, even the best prediction algorithms are some way from creating an accurate protein in one take. “It used to be that 99.99% of the time, it doesn’t work,” AlQuraishi says. “Now it’s more like it only fails 99% of the time.”
That’s partly a problem of logistics, Steinegger says. Computational researchers can run their algorithms over and over until they find something that looks like it will work, and algorithm-design teams such as his own “have new innovations about every three or four months”. Verifying the designed proteins in a biological system, Steinegger estimates, might take two years, by which point the software has already moved on.
This mismatch means that algorithms rarely get the chance to learn from their mistakes. Researchers tend not to publish negative results, even if those failures yielded potentially useful information such as a protein’s cellular toxicity or stability under certain conditions. Barring radical changes in scientific funding models to incentivize such disclosures, researchers must get creative. “It’s extremely challenging to build a team that actually can cover all these facets at once,” Khmelinskaia explains, referring to the bench and computational sides of protein-design research. So, collaboration is a must.
“We’re kind of at this stage where the computer resources and the data are both ready, and that’s why it’s become such a popular field,” Yang says. “The more people work together, the faster they progress.”
Nature 635, 246-248 (2024)
doi: https://doi.org/10.1038/d41586-024-03595-9
This story originally appeared on: Nature - Author:Sara Reardon